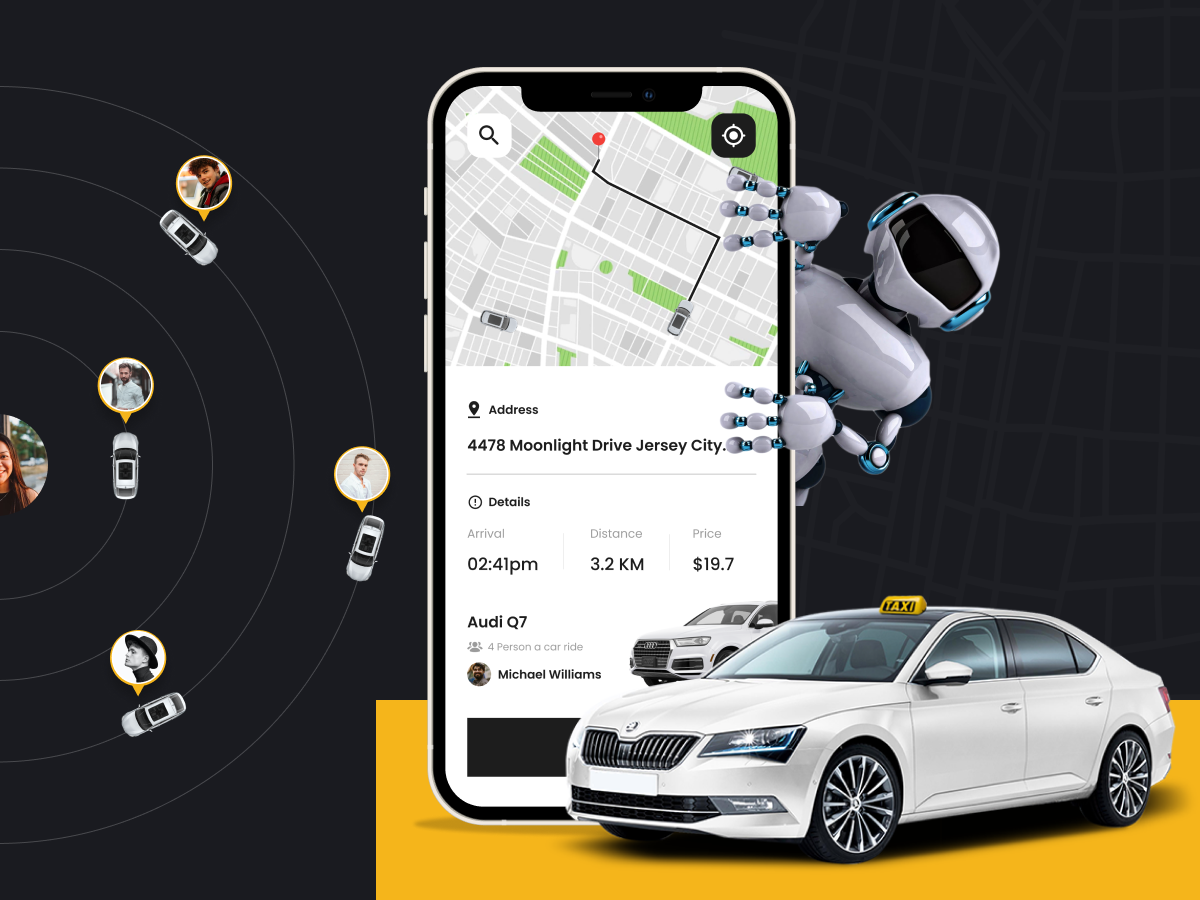
In recent years, artificial intelligence (AI) has emerged as a game changer for many industries, particularly for ride-hailing services such as Uber. Among the various applications of AI, AI-driven dynamic pricing has emerged as one of the most transformative aspects, reshaping the economics of ride-hailing apps. By enabling real-time price adjustments based on a variety of factors, dynamic pricing ensures profitability while improving customer satisfaction. For Uber-like apps, the integration of AI in dynamic pricing models is revolutionizing not only how fares are calculated but also how these apps respond to market changes, supply-demand fluctuations, and external conditions. If you’re wondering how to create an app like Uber, AI-driven dynamic pricing will be a crucial component to consider.
In this blog, we will explore how AI-driven dynamic pricing works, its impact on both users and drivers, and how this technology will shape the future of Uber-like applications.
1. What is AI-Driven Dynamic Pricing?
Dynamic pricing, sometimes referred to as surge pricing or demand-based pricing, is a strategy that adjusts prices in real time based on current market conditions. In the context of Uber-like apps, AI-driven dynamic pricing utilizes sophisticated machine learning algorithms and vast datasets to make real-time decisions on fare prices. These decisions are influenced by a variety of factors, such as:
- Demand for rides: How many users are requesting rides in a given area at a specific time.
- Supply of drivers: The number of available drivers in the vicinity.
- Traffic conditions: Real-time traffic data that impacts the time and distance of a ride.
- Weather conditions: Rain, snow, or extreme weather events often increase demand for rides.
- Events and holidays: Large public events like concerts or holidays can significantly impact demand.
- Competitor pricing: Monitoring competitors’ pricing strategies to stay competitive.
AI models continuously analyze these factors to predict and adjust the optimal fare in real time. This ensures that prices are flexible enough to meet changing conditions, maximize profitability, and still offer value to riders and drivers.
2. How AI-Driven Dynamic Pricing Works in Uber-Like Apps
The core of AI-driven dynamic pricing lies in its ability to process massive amounts of data quickly and make predictive decisions. Here’s a breakdown of how this system works in real time:
a. Data Collection
AI-driven dynamic pricing systems gather data from multiple sources:
- Ride requests: Information about the number of users looking for rides, their locations, and preferred destinations.
- Driver availability: The number of active drivers in an area and their current statuses (busy, idle, or en route).
- Geospatial data: This includes live traffic conditions, weather reports, and significant events happening nearby.
AI algorithms then combine this information to determine how the supply of drivers matches up with demand.
b. Real-Time Processing
AI algorithms process this incoming data using machine learning models that predict demand spikes and drops. For instance, if an AI model detects an influx of users requesting rides near a stadium after a sports event, it anticipates a surge in demand and adjusts prices accordingly to balance the load.
c. Price Optimization
Once the data is processed, the AI adjusts fares dynamically based on multiple objectives:
- Maximizing driver earnings: By increasing prices when demand exceeds supply, drivers are incentivized to accept rides or move to busier areas.
- Maintaining rider satisfaction: AI ensures that price hikes are not extreme, keeping rides affordable even during high-demand periods.
- Ensuring app profitability: Balancing supply and demand ensures a smooth operation for the app, preventing either long wait times for riders or idle time for drivers.
d. Continuous Learning
One of the biggest advantages of AI-driven pricing is its ability to learn and improve over time. Through machine learning, the system can analyze historical pricing and performance data, improving the accuracy of future price predictions.
3. Impact of AI-Driven Dynamic Pricing on Riders
From a rider’s perspective, dynamic pricing introduces both positive and negative changes. While some users may dislike paying more during peak times, AI-driven pricing also ensures that supply meets demand more efficiently.
a. Fair Pricing for Immediate Needs
When demand surges due to inclement weather, public events, or rush hour, AI-driven pricing ensures that available drivers are compensated fairly, while riders still have access to transportation. Although prices might increase, the alternative is waiting longer for a ride or facing a shortage of drivers.
b. Transparency and Predictability
With AI-driven systems, Uber-like apps are able to communicate fare estimates more clearly to riders, offering transparency. Users receive real-time notifications about why prices have surged, whether due to traffic, high demand, or limited driver availability. Over time, these patterns can even become predictable, giving users better insights into when prices will rise or fall.
c. Reduction of Long Wait Times
AI-driven dynamic pricing reduces wait times by efficiently distributing rides among available drivers. Higher prices during peak times encourage more drivers to hit the road, which in turn increases the chances of riders getting a match faster.
d. Personalized Pricing
In the future, AI systems may also enable personalized pricing. Based on users’ past behavior, preferences, and frequency of using the app, AI could offer personalized promotions, discounts, or loyalty rewards, ensuring a more customized and satisfying experience for frequent users.
4. Impact of AI-Driven Dynamic Pricing on Drivers
Drivers, as the other half of the ride-hailing equation, are equally impacted by AI-driven dynamic pricing. This system can help increase their earnings and improve job satisfaction, particularly during high-demand periods.
a. Increased Earnings During Peak Hours
When demand spikes and prices rise, drivers have the opportunity to earn more for each trip. AI-driven pricing ensures that drivers are compensated appropriately for the increased workload during high-demand periods like rush hour or bad weather.
b. Better Route Optimization
Dynamic pricing doesn’t just influence fares. It also provides drivers with insights into the most profitable locations to drive, optimizing their time. For example, if demand is higher in certain neighborhoods, the app can guide drivers to those areas, reducing idle time and increasing their likelihood of getting a fare.
c. Incentivizing Driver Availability
One of the challenges ride-hailing apps face is ensuring enough drivers are on the road during peak times. AI-driven pricing helps tackle this problem by offering higher fares during these periods, which motivates more drivers to be available, balancing supply with demand.
5. The Future of AI-Driven Dynamic Pricing in Uber-Like Apps
AI-driven dynamic pricing is only the beginning of what AI can do for Uber-like apps. As AI technologies become more sophisticated, we can expect further developments that will redefine how these platforms operate.
a. Improved Accuracy with Predictive Analytics
Future AI systems will become even more adept at forecasting demand based on long-term trends and external data. These improvements in predictive analytics will enable ride-hailing companies to preemptively adjust prices and deploy drivers to key locations before demand surges. This could mean better coverage and more consistent pricing for riders.
b. Integration with Autonomous Vehicles
As autonomous vehicles become more prevalent, AI-driven dynamic pricing will extend to fleet management. Ride-hailing apps that operate autonomous vehicles will use AI to balance the cost of running a self-driving fleet with dynamic pricing models, offering lower fares during off-peak times and adjusting based on maintenance or operational factors.
c. AI and Sustainability Goals
With the growing focus on sustainability, AI-driven dynamic pricing could help encourage environmentally friendly choices. For example, ride-hailing apps could offer discounts for carpooling or for choosing electric vehicles, adjusting prices dynamically based on the environmental impact of each trip.
d. New Pricing Models
AI-driven dynamic pricing could also pave the way for new pricing models such as subscription-based pricing for frequent users or micro-pricing, where users can pay based on shorter time intervals, like per-minute rides. These new models can offer flexibility to riders while ensuring steady income streams for drivers.
6. Challenges and Ethical Considerations of AI-Driven Dynamic Pricing
While AI-driven dynamic pricing offers many benefits, it also comes with challenges that ride-hailing companies need to address.
a. Price Gouging Concerns
One of the main criticisms of dynamic pricing is the perception of price gouging, particularly during emergencies or natural disasters. AI-driven systems may unintentionally raise prices to exorbitant levels when demand spikes. Ride-hailing companies will need to establish ethical guidelines and fail-safes to prevent such occurrences.
b. Fairness and Accessibility
Another concern is the potential for dynamic pricing to make rides unaffordable for certain groups, particularly in areas where public transportation is limited. Ensuring equitable pricing that doesn’t disproportionately affect low-income users will be key to the future success of these apps.
c. Data Privacy
AI-driven dynamic pricing relies on collecting vast amounts of data, including geolocation, travel history, and user preferences. Ride-hailing companies will need to prioritize data privacy and security to maintain user trust.
Conclusion
AI-powered dynamic pricing is reshaping the future of Uber-like applications by increasing fee accuracy, balancing supply and demand, and boosting both driver and passenger experiences. While issues such as pricing fairness and data privacy remain, the potential benefits of this technology are substantial. As AI continues to evolve, we can expect even more innovation in the ride-hailing industry, from improved predictive analytics to the integration of autonomous vehicles and sustainable practices. The future of dynamic pricing will not only change the way we travel, but also revolutionize the economics of transportation altogether.